Machine Learning and Artificial Intelligence proponents are convinced – and spend a lot of time trying to convince others – that they will disrupt and revolutionize the current “broken” AML regime. Among other targets within this broken regime is AML alert generation and disposition and reducing the false positive rate (more on false positives in another article!). The result, if we believe the ML/AI community, is a massive reduction in the number of AML analysts that are churning through the hundreds and thousands of alerts, looking for the very few that are “true positives” worthy of being labelled “suspicious” and reported to the government.
But is it that simple? Can the job of AML Analyst be eliminated or dramatically changed – in scope and number of positions – by machine learning and AI? Much has been and continues to be written about the impact of artificial intelligence on jobs. Those writers have categorized jobs along two axes – a Repetitive-to-Creative axis, and an Asocial-to-Social axis – resulting in four “buckets” of jobs, with each bucket of jobs being more or less likely to be disrupted or even eliminated:
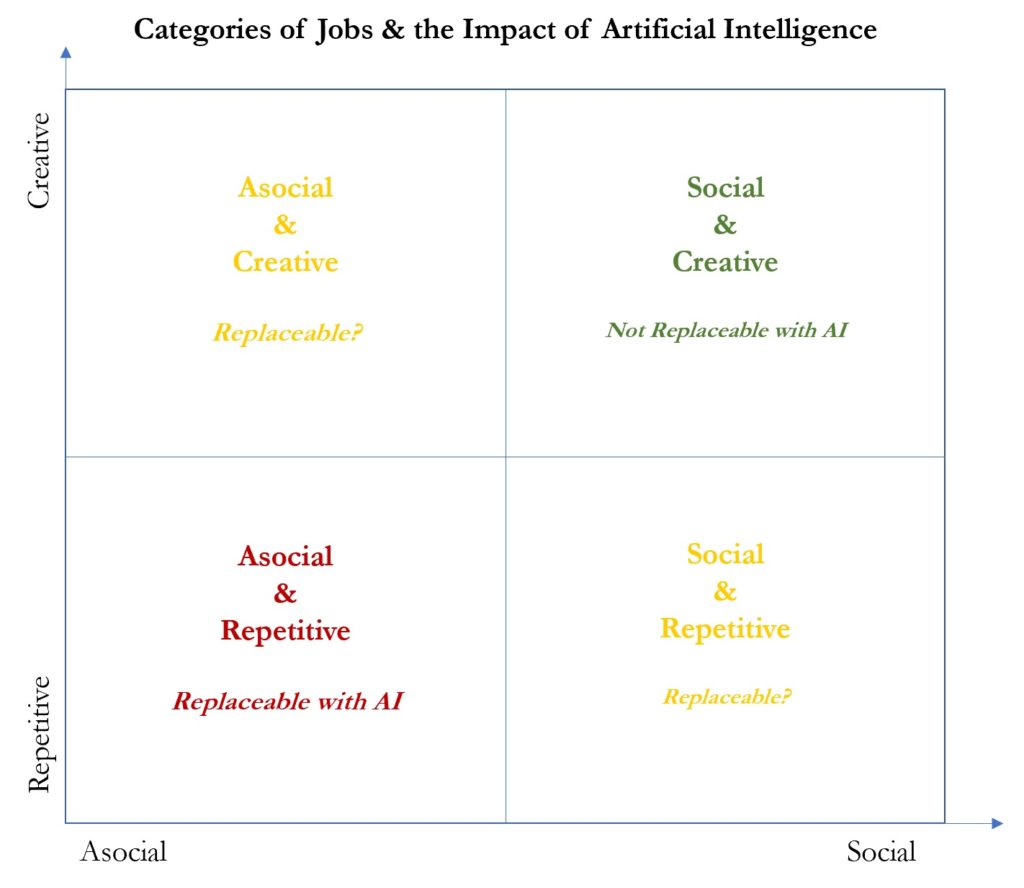
A good example is the “Social & Repetitive” job of Bartender. Bartenders spend much of their time doing very routine, repetitive tasks: after taking a drink order, they assemble the correct ingredients in the correct amounts, and put those ingredients in the correct glass, then present the drink to the customer. All of that could be more efficiently and effectively done with an AI-driven machine, with no spillage, no waste, and perfectly poured drinks. So why haven’t we replaced bartenders? Because a good bartender has empathy, compassion, and instinct, and with experience can make sound judgments on what to pour a little differently, when to cut-off a customer, when to take more time or less with a customer. A good bartender adds value that a machine simply can’t.
Another example could be the “Asocial & Creative” (or is it “Social & Repetitive”?) job of an AML Analyst. Much of an AML Analyst’s time is spent doing very routine, repetitive tasks: reviewing the alert, assembling the data and information needed to determine whether the activity is suspicious, writing the narrative. So why haven’t we replaced AML Analysts? Because a good Analyst, like a good bartender, has empathy, compassion, and instinct, and with experience can make sound judgments on what to investigate a little differently, when to cut-off an investigation, when to take more time or less on an investigation. A good Analyst adds value that a machine simply can’t.
Where AI and Machine Learning, and Robot Process Automation, can really help is by flipping the three currently inefficient AML ratios:
- The False Positive Ratio – the currently accepted, but highly axiomatic and anecdotal, ratio is that 95% to 98% of alerts do not result in SARs, or are “false positives” … although no one has ever boldly stated what an effective or acceptable false positive rate is (even with ROC curves providing some empirical assistance), perhaps the ML/AI/RPA communities can flip this ratio so that 95% of alerts result in SARs. If they can do this, they can also convince the regulatory community that this new ratio meets regulatory expectations (because as I’ll explain in an upcoming article, the false positive ratio problem may be more of a regulatory problem than a technology problem).
- The Forgotten SAR Ratio – like false positive rates, there are anecdotes and some evidence that very few SARs provide tactical or strategic value to law enforcement. Recent Congressional testimony suggests that ~20% of SARs provide TSV (tactical or strategic value) to law enforcement … perhaps the ML/AI/RPA communities can help to flip this ratio so that 80% of SARs are TSV SARs. This also will take some effort from the regulatory and law enforcement communities.
- The Analysts’ Time Ratio – 90% of an AML Analyst’s time can be spent simply assembling the data, information, and documents needed to investigate a case, and only 10% of their time thinking and using their empathy, compassion, instinct, judgment, and experience to make good decisions and file TSV SARs … perhaps the ML/AI/RPA communities can help to flip this ratio so that Analysts spend 10% of their time assembling and 90% of their time thinking.
We’ve seen great strides in the AML world in the last 5-10 years when it comes to applying machine learning and creative analytics to the problems of AML monitoring, alerting, triaging, packaging, investigations, and reporting. My good friend and former colleague Graham Bailey, now at Quantifind, designed and deployed ML and AI systems for AML as far back as 2008-2009. The folks at Verafin, easily the best financial crimes software company on the planet, have deployed cloud-based machine learning tools and techniques to over 2,000 banks and credit unions.
I’ve outlined three rather audacious goals for the machine learning/artificial intelligence/robotic process automation communities:
- The False Positive Ratio – flip it from 95% false positives to 5% false positives
- The Forgotten SAR Ratio – flip it from 20% TSV SARs to 80% TSV SARs
- The Analysts’ Time Ratio – flip it from 90% gathering data to 10% gathering data
Although many new AML-related jobs are being added – data scientist, model validator, etc. – and many existing AML-related jobs are changing, I am convinced that the job of AML Analyst will always be required. Hopefully, it will shift over time from being predominantly that of a gatherer of information and more of a hunter of criminals and terrorists. But it will always exist. If not, I can always fall back on being a Bartender. Maybe …